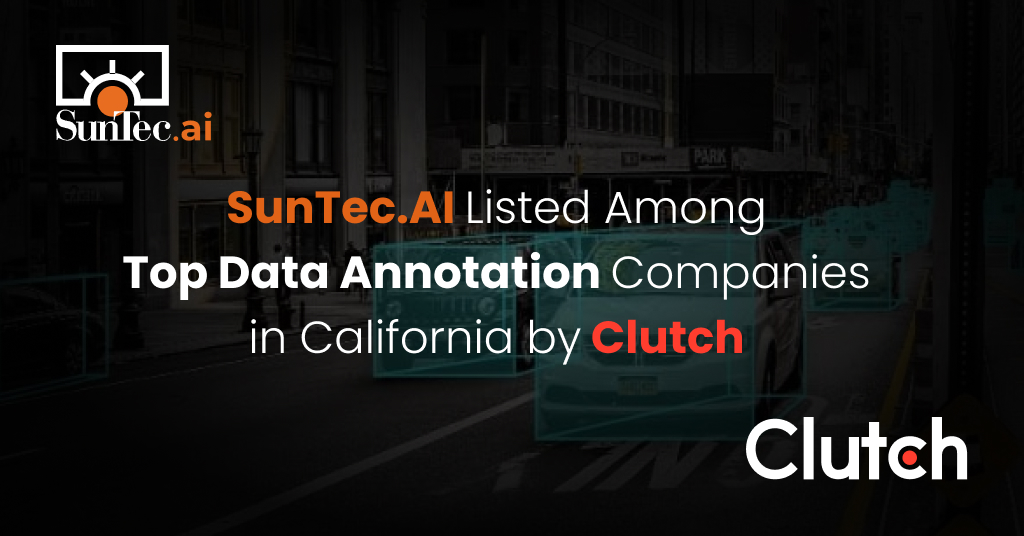
We are delighted to be ranked as one of the leading companies for data annotation services in California by the renowned B2B review and rating platform Clutch. Getting recognized for our data labeling services by a platform like Clutch, which has verified client reviews for 280K+ global providers, is a big achievement. This accolade is a testament to the hard work and dedication of our talented team, as well as the trust and support we’ve received from our valued clients.