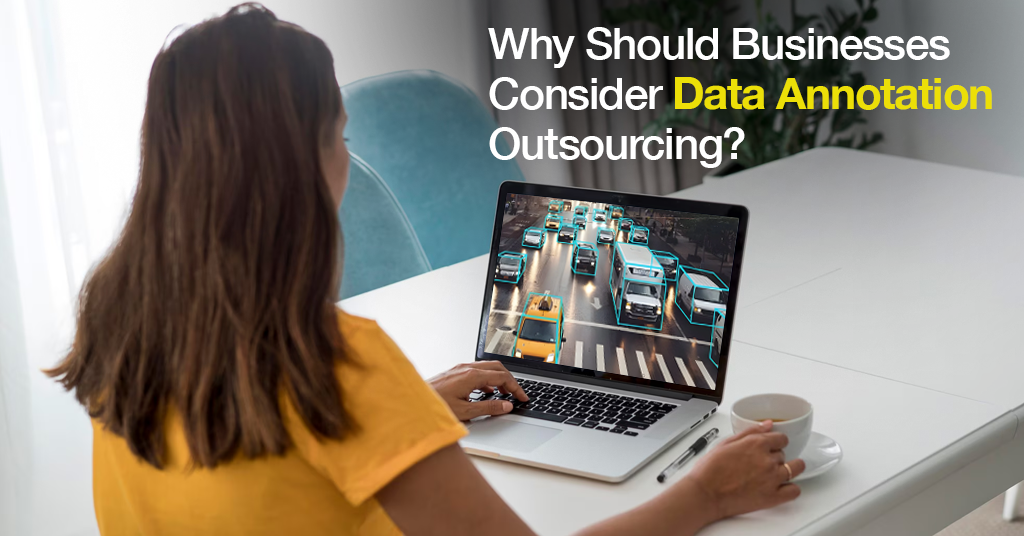
Remember Walmart’s major announcement in 2019 about deploying autonomous floor scrubbers? These machines were designed to navigate and clean floors without human interference. However, there was a problem—the scrubbers frequently collided with obstacles. The issue stemmed from incomplete and inaccurate image annotations. The AI model wasn’t trained to recognize all types of obstacles in the store, leading to repeated errors and damage. So, what did Walmart do? They addressed this issue by feeding their AI model with comprehensive data comprising of more annotated images of the store environment. This enabled the scrubbers to move effectively.
This example highlights the critical role of accurate data annotation in the success of AI projects. However, as the complexity and volume of data increases, not all businesses have the resources or expertise to handle data annotation effectively. That is why most companies today prefer outsourcing data annotation services, which allows them to focus on core business operations while the service provider can handle this process. If you are also struggling to manage data annotation in-house, this blog will help you understand why you should consider outsourcing for improved scalability and operational efficiency.
Table of content
The Need for Scalable Data Annotation Solutions
Unstructured data might not be of much use to companies for training AI and ML models without data annotation. The process helps categorize and label various elements or objects in the datasets based on predefined rules to help AI/ML models learn from them and recognize patterns, classify information, and make accurate predictions when deployed. With the increasing volume, complexity, and velocity of data, the demand for scalable data annotation solutions is on the rise. These solutions empower organizations to:
1. Handle Large Volumes of Data
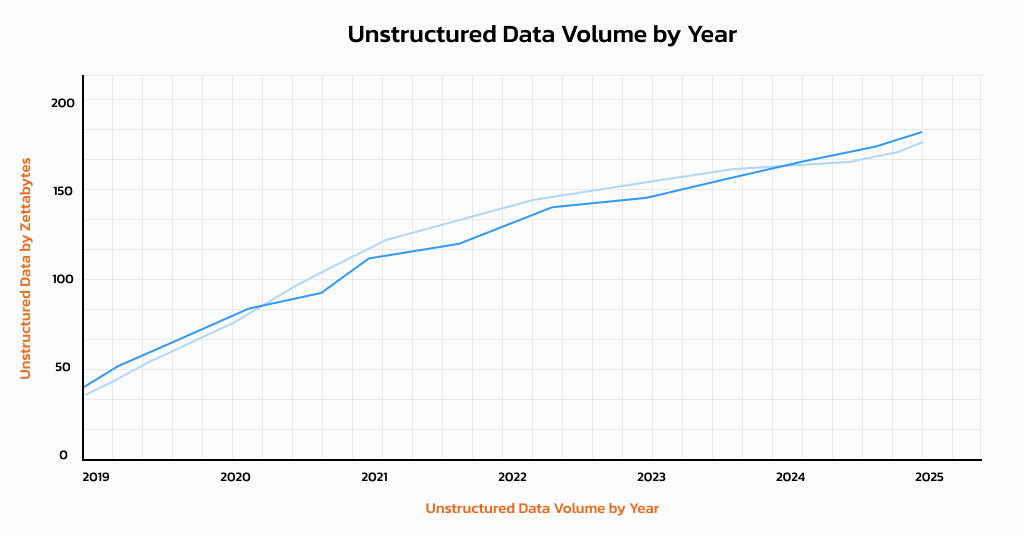
Out of 175 zettabytes of global data predicted to be available by 2025, 80% will be unstructured. When dealing with such data at a growing volume, one needs systems that can integrate new data types and annotation requirements. Scalable annotation solutions can seamlessly handle growing volumes of data through custom workflows and real-time quality check capabilities. Leveraging such solutions, businesses can label vast amounts of data efficiently without compromising quality.
For example, Netflix needs to categorize and label enormous amounts of video content to provide personalized recommendations. Each show or movie must be tagged with attributes like genres, themes, language, mood, and cast details to ensure users receive relevant suggestions. As Netflix’s library and user base expand, the volume of data grows exponentially. To manage this, the company often employs scalable annotation solutions that combine automated tagging algorithms with human reviewers.
2. Manage Complex Use Cases
Complex AI models, such as NLP and facial recognition systems, often demand detailed, nuanced annotations across diverse datasets. Labeling large volumes of diverse data, including text, images, and videos, can become overwhelming without the right infrastructure. Scalable data annotation solutions address this challenge by providing the flexibility to handle increasing data volumes and varying annotation needs efficiently. These solutions enable businesses to maintain consistency and quality across datasets, even as the complexity of use cases grows, ensuring their AI models are trained on accurate and relevant data.
For example, Waymo collects vast amounts of data on a daily basis from its camera and sensors related to surroundings, like the position of vehicles and pedestrians. This data can sometimes have nuanced scenarios like jaywalking, road obstruction, and construction cones, which require detailed labeling. Scalable data solutions can be used here to accurately label these nuanced scenarios and train their AI algorithms to make precise decisions in real-world conditions.
3. Enable Continuous Model Training
Scalable annotation supports iterative model training by providing continuous data feeds to refine and update models as new challenges or edge cases arise. This adaptability allows AI systems to evolve, making them more effective in handling real-world variability and ensuring consistent performance across use cases.
For example, Tesla’s Autopilot, an AI driving assistance system, often mines Tesla cars for visual data to feed its training models. Tesla cars have eight cameras that gather real-time data on traffic, pedestrians, and obstacles. The data is annotated and added to the training set to improve model performance.
4. Ensure Cost-Efficiency
High-quality, scalable annotation ensures better model performance, reducing the need for repeated iterations and costly retraining cycles. This is especially important for startups and small businesses that do not have the funds for rework.
For example, eCommerce startups like Alibaba use scalable hybrid annotation methods by leveraging automated tools and human intervention. The approach reduces costs and provides accurate labels for millions of product listings for search optimization.
Thus, the need for scalable data annotation is undeniable for AI projects. As the data volume and complexity go one step ahead, most companies scale in-house data annotation. However, this raises a question: Is scaling data annotation in-house truly sustainable, or does it call for alternative approaches? Let’s find out!
Challenges Of Scaling In-House Data Annotation
In-house data annotation teams are often in a dilemma – managing both quality and quantity when working on large-scale AI projects. Every dataset has millions of objects that must be labeled correctly so the AI model can learn and provide precise results. When AI projects scale, teams often find it challenging to maintain the same level due to the following reasons:
1. Annotation Fatigue
Annotators repeatedly perform the same tasks, which include outlining objects, classifying images, or transcribing text on a dataset. As AI projects scale, the volume of data and complexity of use cases grow, significantly increasing the workload on annotators. This escalation can result in reduced attention to detail and a rise in data inconsistencies. For companies, this poses challenges in meeting deadlines and upholding strict labeling standards.
2. Unclear Annotation Guidelines
Labeling large volumes of unstructured data in-house becomes challenging when the annotation guidelines aren’t clear, leading to a lack of standardization in labeling. For instance, a person might label ‘car’ as ‘vehicle.’ The varying annotation styles among members can lead to skewed models toward false positives and negatives and increased project turnaround times.
3. Lack of Technological Infrastructure
When datasets are small, teams can work with a few manual annotators who can label the data. However, as data grows in terms of complexity and volume, companies need to revamp their strategy.
The projects now demand AI-assisted annotation tools with pre-annotation features to increase efficiency. Businesses that lack the required infrastructure can face difficulty in handling large volumes of data for annotation. For example, LiDAR data labeling for autonomous vehicles often requires specialized tools to improve project workflows and meet deadlines.
4. Compliance with Data Security Guidelines
Data annotation involves dealing with sensitive information like medical records and financial transactions. Such sensitive data is often transferred between multiple teams, which increases the chances of unauthorized access or data breaches. To ensure that data remains protected and to comply with regulations such as HIPAA, GDPR, CCPA, and DPA, businesses must maintain robust data security standards when working on large-scale AI projects, which is critical but challenging at the same time.
Companies risk regulatory penalties without proper encryption, data masking, or access protocols. For example, failing to comply with GDPR can result in a 20 million euro fine or 4% of the business’s total annual turnover worldwide. In-house teams may struggle to build and maintain workflows that meet these stringent security requirements, adding to the complexity of scaling operations.
5. Time-Consuming and Cost-Intensive
IBM’s CEO, Mr. Arvind Krishna, once said that 80% of the work in an AI project involves collecting and preparing data. This often involves costs and time with respect to training teams, quality assurance, and rework for companies. Because of this, some businesses give up on their AI projects before they even reach deployment. And almost 80% of AI projects fail, nearly double the failure rate of corporate IT projects a decade ago.
To summarize, in-house data annotation is challenging as it affects operational efficiency and makes scalability difficult. To address these issues, most companies today prefer to outsource data annotation.
Why Should You Outsource Data Annotation?
In the last few years, companies have adopted outsourcing solutions for data annotation projects. This is evident from the fact that the outsourced data annotation market is expected to grow at a CAGR of 25.2% from 2024 to 2030. As more and more companies realize the need for high-quality labeled data, they outsource data labeling to reliable service providers to gain the following benefits:
1. Cost-Efficiency
Building and maintaining a dedicated internal team for data annotation team can be expensive as companies have to cover salaries, recruitment costs, and training costs. By outsourcing, companies can do away with these overhead expenses as they need to pay on a project basis.
2. Domain Expertise
Outsourcing data annotation provides access to skilled annotators with domain-specific knowledge, which is critical for accurate labeling, especially in healthcare, autonomous vehicles, and retail. For example, annotators specializing in medical imaging can correctly label anomalies in CT scans to improve diagnostic AI models.
3. Access to Advanced Tools and Technologies
Outsourcing data annotation often gives companies access to industry-leading tools and technologies they otherwise might not have. These tools reduce repetitive tasks and manual effort, thus speeding up the process.
4. Risk Management
Maintaining data integrity is the topmost priority of AI data annotation providers. Most data annotation companies follow strict non-disclosure agreements and implement robust data security measures such as data encryption protocols, authentication, and access controls to comply with regulations like HIPAA and GDPR.
Selecting the Right Outsourcing Partner for Scalable Data Annotation
Once you have decided to outsource data annotation services, the next factor is selecting the right service provider. Here is what you should look for:
1. Industry Experience
Look for a data annotation company specializing in handling domain-specific data. The company should understand Natural Language Processing (NLP), image transcription, video annotation, and other subfields as per your needs.
2. Cost Structure
Every outsourcing partner has a different pricing model. So, you should always compare prices and services among various providers before you outsource data labeling. Ensure they have transparent pricing, no hidden costs, and a balance between affordability and quality.
3. Expertise in Diverse Tools
Ensure the outsourcing partner uses advanced AI annotation tools and is proficient in various techniques, like semantic segmentation, 3D point cloud annotation, or pixel-level annotation. This can improve speed and consistency across large datasets when scaling your AI and ML projects. Data annotation service providers should supplement this with the humans-in-the-loop (HITL) approach to address ambiguous cases that AI cannot handle on its own.
4. Data Security
Confirming that the provider adheres to data protection regulations like GDPR, HIPAA, or CCPA is essential. A good data labeling service provider should have ISO and HIPAA certifications for data security or must follow practices like secure workflows and encryption protocols for managing sensitive data.
5. Scalability
Scalability is vital when working with large datasets. When choosing an outsourcing partner, ensure they have enough resources to take on your project and follow delivery timelines.
Conclusion
In-house data annotation often presents substantial challenges, including scalability issues, inconsistency across datasets, and resource crunch. These obstacles can delay project timelines, increase costs, and even lead to failed AI/ML initiatives. All these challenges can be addressed by outsourcing data annotation services. Reliable service providers help meet the demands of each project and industry through their expertise in diverse labeling techniques, providing you with accurate & high-end training datasets for superior AI/ML outcomes.