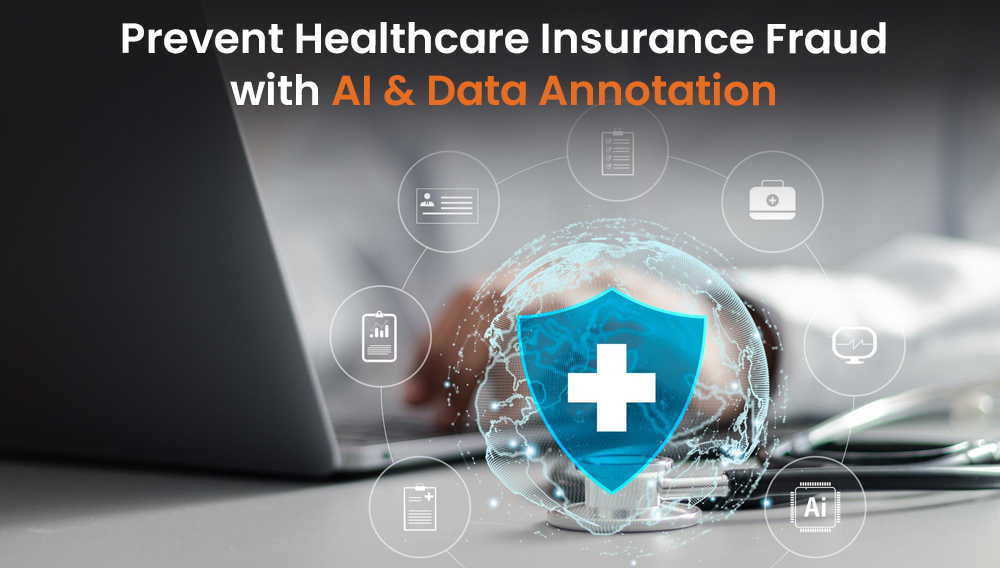
Insurance fraud is one of the most prevalent issues for healthcare providers, resulting in tens of billions in annual revenue losses, as reported by the National Health Care Anti-Fraud Association (NHCAA) in its last publicly available survey. The inefficiencies of manual claims processing only worsen the problem, highlighting the urgent need for innovative solutions. Fortunately, AI and machine learning models have revolutionized the industry, enabling healthcare insurers to detect and prevent fraud with remarkable efficiency and precision. Let’s explore the role of AI in the insurance industry and how it helps healthcare providers mitigate financial losses and manage claims effectively.